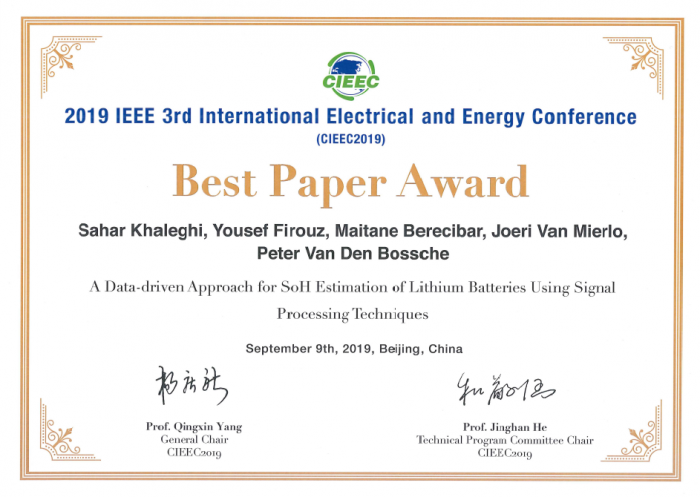
On 7/8/9 September 2019 Sahar Khaleghi of the University of Brussels presented the paper “A Data-driven Approach for SoH Estimation of Lithium Batteries Using Signal Processing Techniques” at the 3rd international electrical and energy conference in Bejing, China.
The presentation was held in the Articificial intelligent panel, where about 50 people attended.
Introduction of the paper:
We’re getting to the end of the oil age very quickly and the renewable energy revolution has taken off around the world. Many companies in the automotive industry have launched initiatives to significantly accelerate their development of electric cars. The success of electric vehicles depends largely on their energy storage system. lithium-ion batteries currently feature the best properties to meet the wide range of requirements specific to automotive applications high energy density long lifetime good power capabilities and low cost. However, the safety and reliability of lithium-ion batteries can be problematic.
Battery health degradation rely on the conditions in which they operate. So, one of the big challenges in electric mobility is battery health diagnosis under a real operating condition in which a variety of stress factors can come into play.
Moreover, precise SoH estimation of lithium ion batteries in electric vehicles boils down how to find proper health indicators whose behaviors change in a predictable way as the battery degrades. Our research attempts to address aforementioned challenges using signal processing and machine learning techniques. The results reveal the proposed approach is capable of estimating SoH of the battery with low computational costs and a relative error of less than 1 %.